Synthetic Data Is a Dangerous Teacher
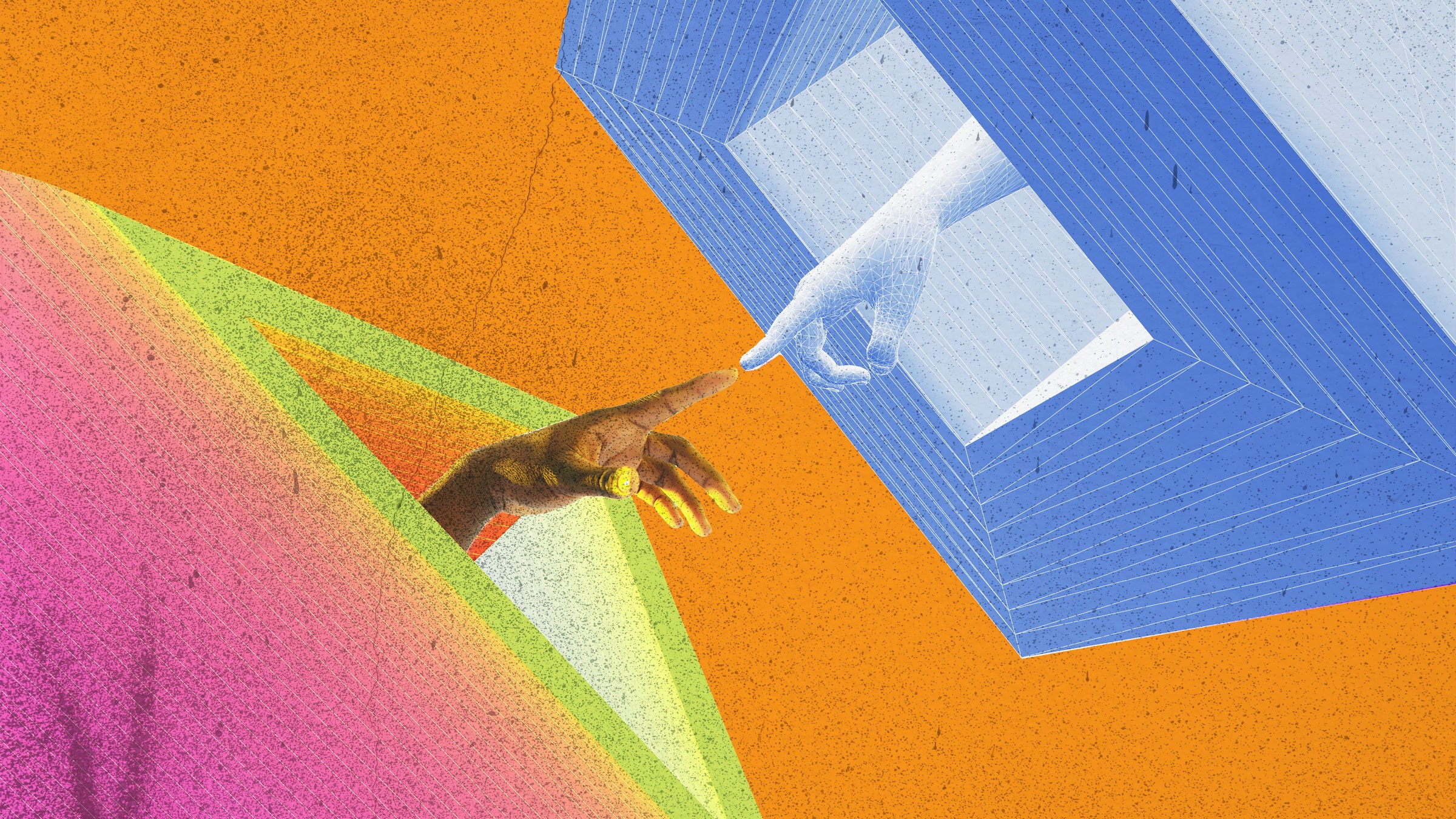
Synthetic Data Is a Dangerous Teacher
Synthetic data, generated artificially rather than collected from real-world sources, has been increasingly used in training machine learning models. While synthetic data can be useful in certain scenarios, it can also be a dangerous teacher that perpetuates biases and inaccuracies.
One of the main risks of using synthetic data is that it may not accurately reflect the complexities and nuances of real-world data. This can lead to models that are trained on unrealistic or skewed data, resulting in poor performance when applied to real-world scenarios.
Furthermore, synthetic data can inadvertently reinforce existing biases present in the training data used to generate it. If the original training data contains biases, these biases can be amplified in the synthetic data, leading to models that perpetuate and even exacerbate societal inequalities.
Another issue with synthetic data is the potential for overfitting. In an effort to create diverse and robust training data, synthetic data generators may inadvertently introduce noise or irrelevant patterns that the model learns to rely on, leading to poor generalization and performance on unseen data.
Despite these risks, there are ways to mitigate the dangers of synthetic data. Careful validation and testing of synthetic data against real-world data can help ensure that the synthetic data accurately reflects the underlying distribution and characteristics of the real data.
Additionally, incorporating diversity and fairness considerations into the generation process can help mitigate biases and promote more ethical and accurate models.
In conclusion, while synthetic data can be a powerful tool for training machine learning models, it must be used with caution. By understanding the potential dangers of synthetic data and taking steps to mitigate these risks, we can ensure that our models are more accurate, fair, and robust in real-world applications.